Introduction
We are thrilled to announce that our ground-breaking research on ovarian assessment using deep learning-based 3D ultrasound super-resolution has been cited in a recent publication. This citation highlights the impact of our work in advancing the field of assisted pregnancy technology and improving the accuracy of ovarian volume computation. In this blog post, we will discuss the key findings of our study and how they have contributed to the development of an innovative Harmonic Attention-based U-Net network, HaTU-Net.
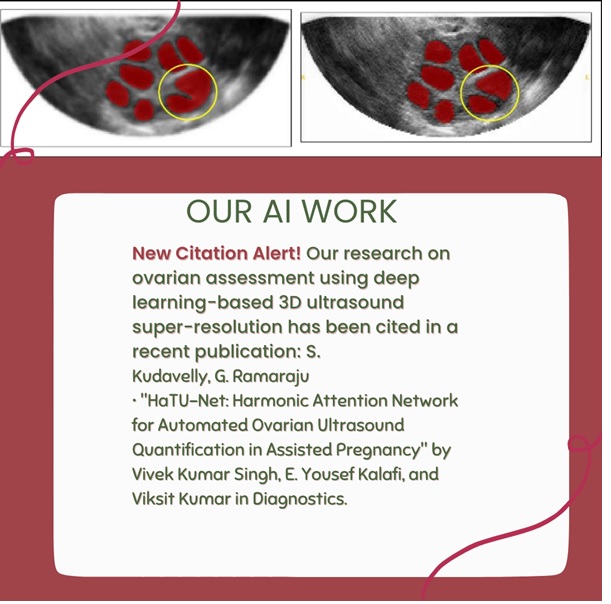
Our Research: Super-Resolving 3D Ultrasound Data for Ovarian Assessment
Ovarian volume assessment is crucial in determining a woman’s reproductive age and diagnosing polycystic ovary syndrome (PCOS). Accurate measurement of ovarian volume and surface assessment is vital for both clinical diagnosis and treatment planning. In our study, we developed a deep learning method for super-resolving 3D ultrasound (US) data along the axial direction to address this challenge.
Our method achieved high PSNR (Peak Signal-to-Noise Ratio) and SSIM (Structural Similarity Index) values, demonstrating a 54% improvement in ovarian volume computation accuracy. Additionally, our approach improved the quality of the 3D rendering of the ovary and reduced the problem of fused follicles in segmentation, proving its viability for clinical diagnostic assessment.
HaTU-Net: A Novel Network for Ovarian Ultrasound Quantification in Assisted Pregnancy
The recent publication citing our work, “HaTU-Net: Harmonic Attention Network for Automated Ovarian Ultrasound Quantification in Assisted Pregnancy” by Vivek Kumar Singh et al., introduces a novel Harmonic Attention-based U-Net network, HaTU-Net. This network aims to provide precise segmentation of the ovary and follicles in ultrasound images. By replacing the standard convolution operation with a harmonic block that convolves the features with a window-based discrete cosine transform (DCT), HaTU-Net offers a significant advancement in ovarian assessment technology.
The Future of Assisted Pregnancy Technology
The citation of our research in this ground-breaking study is an honour and testament to the importance of our work in advancing assisted pregnancy technology. As researchers continue to develop innovative solutions to improve ovarian assessment and diagnosis, we can expect to see significant improvements in treating and managing fertility-related conditions. By combining deep learning methods with state-of-the-art ultrasound technology, we can continue to push the boundaries of assisted pregnancy and contribute to a brighter future for those seeking help with fertility.
Conclusion
We are proud to see our research on deep learning-based 3D ultrasound super-resolution contributing to the ongoing development of assisted pregnancy technology. As more researchers build upon our work and develop new ovarian assessment and diagnosis methods, we look forward to witnessing continued progress in this vital field. Together, we can help make fertility treatments more accurate, effective, and accessible for individuals worldwide.